AC Power Flow Analysis#
Information |
Details |
---|---|
Lead Author |
Hantao Cui |
Learning Objectives |
• Understand power flow equations and their mathematical formulation |
Prerequisites |
• Basic knowledge of power systems |
Estimated Time |
90 minutes |
Topics |
Power flow equations, Newton-Raphson method, Numerical Jacobian calculation, Admittance matrix, Network visualization, Computational efficiency |
Conceptual Overview#
A typical nonlinear equation is the power flow equations. Power flow equations are algebraic equations enforcing the power balancing at each bus. Intuitively, the equations require that, at each bus, the power injection (generation minus load) is equal to the power that leaves the bus through connected lines.
Using the complex power definition, the power flow equations are as simple as:
where \(P_i\) and \(Q_i\) are the real and reactive power injection at bus \(i\), \(V_i\) and \(V_j\) are the voltage at bus \(i\) and \(j\), \(Y\) is the admittance matrix, and
If bus k
has no injection, the corresponding \(P_k\) and \(Q_k\) are zero.
For a system with \(N\) buses, there are \(2N\) real-valued equations. There has to be \(2N\) unkonwns to solve the equations.
As we have learned from power system analysis, there are three types of buses, PQ, PV and Slack. The unknowns are:
Bus Type |
Knowns |
Unknowns |
---|---|---|
PQ |
\(P_i, Q_i\) |
\(V_i, \theta_i\) |
PV |
\(P_i, V_i\) |
\(Q_i, \theta_i\) |
Slack |
\(V_i, \theta_i\) |
\(P_i, Q_i\) |
Worked Example: 3-Bus Power System#
We consider a three-bus system from [1] (Example 3.11). I have taken the liberty to use a 0-based index to align with Python code.
It is a three bus sytem with the following bus parameters:
Bus |
Type |
V |
Pgen |
Qgen |
Pload |
Qload |
---|---|---|---|---|---|---|
0 |
Slack |
1.02 |
– |
– |
0.0 |
0.0 |
1 |
PV |
1.00 |
0.5 |
– |
0.0 |
0.0 |
2 |
PQ |
– |
0 |
0 |
1.2 |
0.5 |
And the line parameters are given as
From |
To |
R |
X |
B |
---|---|---|---|---|
0 |
1 |
0.02 |
0.3 |
0.15 |
0 |
2 |
0.01 |
0.1 |
0.1 |
1 |
2 |
0.01 |
0.1 |
0.1 |
First, let’s make the admittance matrix.
import numpy as np
from scipy.optimize import fsolve
Y = np.zeros((3, 3), dtype=np.complex128)
# Diagonal elements
Y[0, 0] = 1 / (0.02 + 0.3j) + 1 / (0.01 + 0.1j) + 1j * 0.15 / 2 + 1j * 0.1 / 2
Y[1, 1] = 1 / (0.02 + 0.3j) + 1 / (0.01 + 0.1j) + 1j * 0.15 / 2 + 1j * 0.1 / 2
Y[2, 2] = 1 / (0.01 + 0.1j) + 1 / (0.01 + 0.1j) + 1j * 0.1 / 2 + 1j * 0.1 / 2
# Off-diagonal elements
Y[0, 1] -= 1 / (0.02 + 0.3j)
Y[1, 0] -= 1 / (0.02 + 0.3j)
Y[0, 2] -= 1 / (0.01 + 0.1j)
Y[2, 0] -= 1 / (0.01 + 0.1j)
Y[1, 2] -= 1 / (0.01 + 0.1j)
Y[2, 1] -= 1 / (0.01 + 0.1j)
print(Y)
[[ 1.21133795-13.09457417j -0.22123894 +3.31858407j
-0.99009901 +9.9009901j ]
[-0.22123894 +3.31858407j 1.21133795-13.09457417j
-0.99009901 +9.9009901j ]
[-0.99009901 +9.9009901j -0.99009901 +9.9009901j
1.98019802-19.7019802j ]]
We can verify that the result matches the textbook:
# print Y in a polar form (using degrees)
print("Y magnitudes:")
print(np.abs(Y))
print("Y angles:")
print(np.angle(Y, deg=True))
Y magnitudes:
[[13.15048335 3.32595053 9.9503719 ]
[ 3.32595053 13.15048335 9.9503719 ]
[ 9.9503719 9.9503719 19.80124259]]
Y angles:
[[-84.71478916 93.81407483 95.71059314]
[ 93.81407483 -84.71478916 95.71059314]
[ 95.71059314 95.71059314 -84.26061502]]
We can move on and write the power flow equations. To conform with the standard form of f(x) = 0
, the power injections are subtracted from both sides.
def pf_3bus(x):
# we can make the unknowns to be
# x = [P0, Q0, theta1, Q1, theta2, V2]
# where theta is in radians and V is in per unit
V = np.array([1.02, 1.00, x[5]])
theta = np.array([0.0, x[2], x[4]])
Vc = V * np.exp(1j * theta)
# calculate the power injection into the network
# note that power leaves the bus into the network
S = np.diag(Vc) @ np.conj(Y @ Vc)
# power leaving each bus via the lines
# minus power injection at each bus shall equal to 0
P = np.real(S) - np.array([x[0], 0.5, -1.2])
Q = np.imag(S) - np.array([x[1], x[3], -0.5])
return np.concatenate([P, Q])
initial_guess = [0, 0, 0, 0, 0, 1.0]
sol, infodict, ier, mesg = fsolve(pf_3bus, initial_guess, full_output=True)
print(sol)
[ 0.70867913 0.28056964 -0.01009172 -0.04462179 -0.06351473 0.98158465]
This result is exactly the same as the results from the textbook.
Key Formulation Notes#
If you have some impressions from a Power System Analysis course, you may remember the lengthy derivations of the power flow equations and its Jacobian matrix. In fact, this formulation comes much simpler than the textbook ones, so it can be interesting to understand why both formulations work.
Exercise
You may also have many questions about the formulation, such as
Why are the P&Q at a slack bus and the Q at a PV bus included as variables, resulting in six variables for three buses?
Following up on the previous question, why do we include two equations at the slack bus and one reactive power equation at the PV bus?
Why are the variables not ordered in the typical way, namely, unknown phase angles and then unknown voltage magnitudes, yet it solves?
Following up on the previous question, why is that ordering the convention (as written in textbooks and implemented in many programs)? Is that still relevant?
Please work on these exercise questions.
Hints
They are dependent variables and can be either included in the full problem (to be solved simultaneously), or removed from the problem but evaluated when other unknowns are found.
The number of variables must equal the number of (independent) equations.
Variables and equations can be in arbitrary order.
Grouping variables and equations will help create structural patterns in the Jacobian matrix.
Calculating Jacobian Numerically#
def numerical_jacobian(func, x, eps=1e-6):
"""Calculate the Jacobian matrix using finite differences."""
n = len(x)
f0 = func(x)
m = len(f0)
J = np.zeros((m, n))
for j in range(n):
# Create a copy of x with a small perturbation in the j-th element
x_perturbed = x.copy()
x_perturbed[j] += eps
# Calculate the perturbed function values
f1 = func(x_perturbed)
# Compute the j-th column of the Jacobian
J[:, j] = (f1 - f0) / eps
return J
# Example: Calculate the Jacobian for our 3-bus power flow problem
x = np.array(
[0.70867913, 0.28056964, -0.01009172, -0.04462179, -0.06351473, 0.98158465]
)
J = numerical_jacobian(pf_3bus, x)
np.set_printoptions(precision=4)
print("Numerical Jacobian matrix (shape):", J.shape)
print(J)
Numerical Jacobian matrix (shape): (6, 6)
[[ -1. 0. -3.3871 0. -9.956 -0.3669]
[ 0. 0. 13.1392 0. -9.7567 -0.46 ]
[ 0. 0. -9.6529 0. 19.483 0.7212]
[ 0. -1. 0.1915 0. 0.3601 -10.1427]
[ 0. 0. -0.7113 -1. 0.4515 -9.9397]
[ 0. 0. 1.4894 0. -3.1079 18.8298]]
Applying to Large Test Systems#
The implementation above is elegant due to the very few lines of code. However, without providing the Jacobian matrix, computational speed challenges are expected.
We will use the ANDES software to load the IEEE 300-bus system.
The code below downloads the data from the MATPOWER repository, stores it in a temporary file, and loads it using ANDES.
import os
import tempfile
import urllib.request
# Test case name -- change it for testing
case_name = "case300.m"
def download_to_temp(case_name):
"""
Download a MATPOWER case to a local temp file.
"""
base_url = "https://raw.githubusercontent.com/MATPOWER/matpower/refs/heads/master/data/"
url = base_url + case_name
# Create a temporary file
with tempfile.NamedTemporaryFile(delete=False, suffix=".m") as temp_file:
# Download the file
urllib.request.urlretrieve(url, temp_file.name)
print(f"File downloaded and saved to {temp_file.name}")
# Verify if the file exists
if os.path.exists(temp_file.name):
print(f"File size: {os.path.getsize(temp_file.name)} bytes")
else:
print("Download failed")
return temp_file
temp_file = download_to_temp(case_name)
File downloaded and saved to /tmp/tmpxsbgymrt.m
File size: 65678 bytes
import andes
from kvxopt import matrix
ss = andes.load(temp_file.name, no_output=True)
The code below builds the admittance matrix and obtains the indices needed to reuse the power residual equations from the above.
Y = matrix(ss.Line.build_y())
class NodalData:
def __init__(self, ss):
nbus = ss.Bus.n
self.P = np.zeros(nbus)
self.Q = np.zeros(nbus)
self.V = np.ones(nbus)
self.theta = np.zeros(nbus)
def set_zero(self):
self.P[:] = 0
self.Q[:] = 0
self.V[:] = 0
self.theta[:] = 0
ndata = NodalData(ss)
The following boilerplate code takes care of the indexing machinery. It is needed due to the ANDES data structure, where PQ, Slack and PV generators are stored separately from Bus.
class IndexLoc:
def __init__(self, ss):
self.pq = ss.Bus.idx2uid(ss.PQ.bus.v)
self.pv = ss.Bus.idx2uid(ss.PV.bus.v)
self.slack = ss.Bus.idx2uid(ss.Slack.bus.v)
self.gen = np.concatenate([self.pv, self.slack])
self.non_gen = np.setdiff1d(np.arange(ss.Bus.n), self.gen)
self.non_slack = np.setdiff1d(np.arange(ss.Bus.n), self.slack)
self.shunt = ss.Bus.idx2uid(ss.Shunt.bus.v)
loc = IndexLoc(ss)
Next, we will define the power mismatch equations.
def pf_large(x, ss, ndata, loc):
P, Q, V, theta = ndata.P, ndata.Q, ndata.V, ndata.theta
# Important: clear the injection vector at the beginning of iteration
ndata.set_zero()
# PQ
np.subtract.at(P, loc.pq, ss.PQ.p0.v)
np.subtract.at(Q, loc.pq, ss.PQ.q0.v)
# PV
V[loc.pv] = ss.PV.v0.v
np.add.at(P, loc.pv, ss.PV.p0.v)
# Slack
theta[loc.slack] = ss.Slack.a0.v
V[loc.slack] = ss.Slack.v0.v
# retrieve unknowns from `x`
# Unknowns in `x` are grouped by theta and then V
# Note that the `loc.non_gen` can have random order
# No sorting is needed, because x will be in the same order
theta[loc.non_slack] = x[: len(loc.non_slack)]
V[loc.non_gen] = x[len(loc.non_slack) :]
# shunt elements
np.add.at(P, loc.shunt, ss.Shunt.g.v * V[loc.shunt] ** 2)
np.add.at(Q, loc.shunt, ss.Shunt.b.v * V[loc.shunt] ** 2)
Vc = V * np.exp(1j * theta)
S = np.diag(Vc) @ np.conj(Y @ Vc)
# Power leaving each bus via the lines
# minus the amount of injection shall be equal to 0
Pmis = np.real(S) - P
Qmis = np.imag(S) - Q
mis = np.concatenate([Pmis[loc.non_slack], Qmis[loc.non_gen]])
return mis
Moving on, the initial guess is set to solve the problem as a flat start (ones for voltages and zeros for unknown phase angles).
def get_initial_guess(ss, loc):
return np.concatenate([ss.Bus.a0.v[loc.non_slack],
ss.Bus.v0.v[loc.non_gen]])
initial_guess = get_initial_guess(ss, loc)
sol, infodict, ier, mesg = fsolve(
pf_large, initial_guess, args=(ss, ndata, loc), full_output=True
)
print(mesg)
The solution converged.
print(f"Voltage magnitude vector:\n{ndata.V}")
print(f"Voltage phase angle vector:\n{ndata.theta}")
Voltage magnitude vector:
[1.0284 1.0353 0.9971 1.0308 1.0191 1.0312 0.9934 1.0153 1.0034 1.0205
1.0057 0.9974 0.9977 0.9992 1.0344 1.0316 1.0649 0.9819 1.001 0.9752
0.9963 1.0501 1.0057 1.0234 0.9986 0.975 1.0248 1.0415 0.9757 1.0012
1.0205 1.0205 1.0537 1.0219 1.0295 1.045 1.0009 1.0088 1.0217 1.0346
0.9779 1.0021 1.0475 1.0255 0.9981 0.9962 1.0052 1.0152 1.0335 0.9918
0.9789 1.0248 0.9907 1.016 0.9583 0.948 0.9627 0.9513 0.9794 0.9696
0.9776 0.9965 0.9632 0.9838 0.99 0.982 0.9872 1.0342 1.025 0.9871
0.9908 0.992 1.0153 1.0318 1.0274 1.052 1.052 0.9929 1.0182 1.
0.9894 1.006 1.0007 1.0288 0.9957 1.0223 1.0095 0.99 0.9753 0.9732
0.9745 0.9702 0.9768 0.9603 1.0249 0.9348 0.9299 1.0435 0.9584 0.9871
0.9728 1.0006 1.0233 1.0103 0.9978 1.0001 1.0024 1.0028 1.0191 0.9861
1.0046 1.002 1.0221 1.0193 1.0476 1.0471 1.055 1.0117 1.043 1.051
1.0155 1.0435 1.0161 1.0081 1.0528 1.0528 1.0577 1.0735 0.9869 1.0048
1.0535 1.0435 0.9664 1.0177 0.963 0.9845 0.9987 0.9866 0.9998 1.0361
0.9919 1.0411 0.984 1.0002 0.9973 0.9715 1.0024 0.9879 0.929 0.9829
1.0245 0.9837 1.0622 0.9731 1.0522 1.0077 0.9398 0.9699 0.9793 1.0518
1.0446 0.9716 1.0386 1.0522 1.065 1.065 1.0533 1.0027 1.0551 1.0435
0.9375 0.9982 1.049 1.0359 0.974 0.9925 1.015 0.9543 0.9562 0.974
0.9908 1.0034 0.9667 0.9855 1.0038 1.0185 0.9994 1.0048 0.9805 1.0018
1.0133 1.01 0.9919 0.9866 0.9751 1.0215 1.0076 1.0554 1.008 1.
1.05 0.9966 1.0003 0.9453 1.018 1. 1.0424 1.0496 1.04 1.0535
1.0415 1. 1.0387 1.0095 1.0165 1.0559 1.01 1. 1.0238 1.05
0.993 1.01 0.9922 0.9711 0.9652 0.9691 0.977 0.9762 1.0205 1.0251
1.0152 1.0146 1.0004 0.9809 0.9749 0.9429 0.9724 0.9605 1.0009 0.9778
0.9583 1.031 1.0129 1.0244 1.0122 0.9695 1.0507 1.0507 1.0323 1.0145
1.0507 1.0507 1.0507 1.029 1.05 1.0145 1.0507 0.9967 1.0212 1.0145
1.0017 0.9893 1.0507 1.0507 1.0145 1.0121 0.9945 0.9884 0.9821 1.012
1.0066 0.9959 1.0024 0.9889 0.9659 0.9757 0.9714 0.9661 0.9669 0.9394
0.9509 0.9366 1.0027 0.957 0.9649 0.9632 0.9463 0.9693 0.9567 0.97
0.9842 1. 0.979 1. 1. 1. 0.9805 0.9853 0.98 1.0405]
Voltage phase angle vector:
[ 0.1056 0.1368 0.1176 0.0839 0.0835 0.1237 0.1097 0.0436 0.0515
0.0252 0.0447 0.0927 -0.008 -0.0823 -0.1481 -0.0444 -0.2269 0.0204
-0.0413 0.0299 -0.0328 0.0704 0.1067 0.0268 -0.0286 -0.0838 -0.2081
-0.1365 -0.4468 -0.3916 -0.1942 -0.2174 -0.0994 -0.2213 -0.1806 -0.1279
-0.2912 -0.3028 -0.255 -0.2028 -0.4028 -0.2799 -0.0511 -0.1411 -0.2055
-0.3054 -0.2818 -0.2115 -0.1377 -0.1027 -0.0903 -0.1647 -0.0586 -0.0172
-0.3056 -0.2244 -0.4606 -0.6116 -0.5195 -0.4775 -0.4478 -0.3816 -0.4611
-0.4334 -0.4181 -0.4351 -0.4317 -0.3259 -0.2979 -0.3086 -0.2472 -0.1346
-0.3628 -0.193 -0.1946 -0.1632 -0.1075 -0.1635 -0.2304 -0.2545 -0.3541
-0.2516 -0.2654 -0.21 -0.3022 -0.2254 -0.2792 -0.3539 -0.4574 -0.4337
-0.5095 -0.4427 -0.5083 -0.2351 -0.2197 -0.0806 -0.0702 0.0919 -0.1513
-0.2188 -0.2489 -0.306 -0.2336 -0.3198 -0.2227 -0.1819 -0.0816 -0.075
0.0988 0.1074 0.0549 -0.0935 -0.1386 -0.1161 0.0287 -0.0236 -0.1091
-0.0605 -0.0582 0.0027 -0.0465 0.0723 -0.0102 -0.0009 0.0773 0.1478
0.0067 0.0931 0.1123 0.0738 0.1631 0.1844 -0.0296 0.1196 0.0916
-0.2064 -0.1972 -0.1696 -0.2173 0.1562 0.3247 0.0525 0.1704 0.4609
0.5292 -0.1188 -0.082 -0.1148 0.0033 -0.1716 -0.1068 -0.2208 -0.0451
-0.1239 0.0833 0.0127 -0.1127 -0.1616 -0.0521 -0.0213 -0.0713 0.1261
-0.1177 -0.0738 0.0397 0.0262 -0.0108 -0.4526 -0.3549 0.2187 -0.1902
-0.478 -0.331 -0.3578 -0.4214 -0.4011 -0.3493 -0.4427 -0.4413 -0.5088
-0.4344 -0.3812 -0.5143 -0.4963 -0.4955 -0.4924 -0.4698 -0.4456 -0.4105
-0.3995 -0.3861 -0.1972 -0.2994 -0.3468 -0.3873 -0.3823 -0.3899 -0.3642
-0.3742 -0.3875 -0.3994 -0.3912 -0.3712 -0.193 -0.3722 -0.4701 -0.3605
-0.3431 -0.2363 -0.3655 -0.3999 -0.4471 -0.3597 -0.362 -0.2638 -0.3633
-0.3605 -0.2718 -0.3465 -0.2831 -0.2992 -0.3293 -0.3457 -0.3577 -0.3724
-0.3709 -0.4316 -0.439 -0.4066 -0.3452 0.0274 -0.0374 -0.3073 -0.2385
-0.409 -0.5969 -0.6538 -0.5058 -0.4058 -0.4863 -0.5005 -0.2924 0.0699
-0.1295 -0.2631 -0.4297 0.1901 0.2196 0.2418 0.0888 0.2037 -0.1809
0.109 0.2215 0.0388 -0.2409 0. -0.1292 -0.0582 0.0363 0.1031
-0.4404 0.3338 0.0497 0.6135 -0.1945 -0.3241 -0.3302 -0.3324 -0.1958
-0.294 -0.3141 -0.2972 -0.3278 -0.3683 -0.3325 -0.3645 -0.3494 -0.348
-0.4134 -0.3953 -0.4186 -0.3524 -0.3846 -0.3818 -0.3754 -0.4039 -0.3559
-0.3742 -0.3601 -0.3318 -0.3367 -0.2992 -0.3059 -0.1174 -0.1298 -0.3421
-0.3334 -0.3325 -0.3134]
It is worth mentioning that the solution above for the IEEE 300-bus system matches the ANDES and MATPOWER solution. We can see the code implementation is straightforward. By using SciPy’s fsolve
function, the power flow problem is formulated as a general root-finding problem and readily solved.
Performance Remarks on the Numerical Jacobian#
With this example, we also illustrate that solving large power flow cases without providing the Jacobian matrix is computationally inefficient.
%timeit fsolve(pf_large, initial_guess, args=(ss, ndata, loc))
267 ms ± 84.6 ms per loop (mean ± std. dev. of 7 runs, 1 loop each)
It reports around 200 ms to solve the 300-bus power flow. The following reports the solution time using ANDES:
andes.config_logger() # show logging output
ss.PFlow.run();
-> System connectivity check results:
No islanded bus detected.
System is interconnected.
Each island has a slack bus correctly defined and enabled.
-> Power flow calculation
Numba: Off
Sparse solver: KLU
Solution method: NR method
Power flow initialized in 0.0036 seconds.
0: |F(x)| = 10.51482497
1: |F(x)| = 2.585704245
2: |F(x)| = 0.3536420223
3: |F(x)| = 0.008448363916
4: |F(x)| = 4.64692361e-06
5: |F(x)| = 1.376843084e-12
Converged in 6 iterations in 0.0129 seconds.
The logging output shows that ANDES takes about 10 ms to solve the same case.
There are two main reasons:
Divided difference requires 2*N evaluations of the residual function, where
N
is the number of rows (or columns) of the matrixThe Jacobian matrix from the divided difference is formulated as a dense matrix.
The second reason needs more elaboration. Divided difference has no information about the sparsity pattern by just having a handle to the residual function, so the initial result must be stored as a dense matrix. The solver can choose to convert it to a sparse matrix or not, but such a conversion has memory cost and must be done for each step. For small to medium sized systems, the conversion overhead may outweight the gain.
Sparsity Pattern in the Jacobian Matrix#
The IEEE 300-bus system is large enough to demonstrate the sparsity of the Jacobian matrix. In our problem formulation, the unknown variables (theta and V) are grouped. The residual equations are also grouped by active and reactive power residuals. Therefore, the Jacobian matrix is expected in a block-structured form of:
The Jacobian matrix will be visualized next. First, let’s retrieve the Jacobian matrix from the last step of the iterations by fsolve
:
fjac = infodict["fjac"]
# Apply threshold to remove small values
thres = 1e-3
fjac_thresholded = fjac.copy()
fjac_thresholded[np.abs(fjac_thresholded) < thres] = 0
Note on the threshold
The threshold of 1e-3 is used to remove small values from the Jacobian matrix. These small values can come from rounding errors in divided differences or small values as a result of system parameters. The threshold value is somewhat arbitrary and should not be set too large.
The following script plots the Jacobian matrix - the non-zero elements are in black, and the zeros are in white. The horizontal axis correspond to the variables, and the vertical axis correspond to the residual equations.
%matplotlib inline
import matplotlib.pyplot as plt
fig, ax = plt.subplots(figsize=(8, 8))
# Plot the sparsity pattern
ax.spy(fjac_thresholded, markersize=0.5, color='black')
# Add partitioning lines to show the submatrices (P-θ, P-V, Q-θ, Q-V)
n_theta = len(loc.non_slack)
n_v = len(loc.non_gen)
ax.axhline(y=n_theta, color='r', linestyle='--', alpha=0.7, linewidth=2)
ax.axvline(x=n_theta, color='r', linestyle='--', alpha=0.7, linewidth=2)
# Add labels for the submatrices
ax.text(n_theta/2, -10, r'$\theta$', fontsize=14, ha='center')
ax.text(n_theta + n_v/2, -10, r'$V$', fontsize=14, ha='center')
ax.text(-10, n_theta/2, r'$P$', fontsize=14, va='center')
ax.text(-10, n_theta + n_v/2, r'$Q$', fontsize=14, va='center')
# Add titles and labels
ax.set_title(f'Jacobian Matrix Sparsity Pattern (values < {thres} set to zero)', fontsize=14)
ax.set_xlabel('Variable index', fontsize=12)
ax.set_ylabel('Equation index', fontsize=12)
plt.tight_layout()
plt.show()
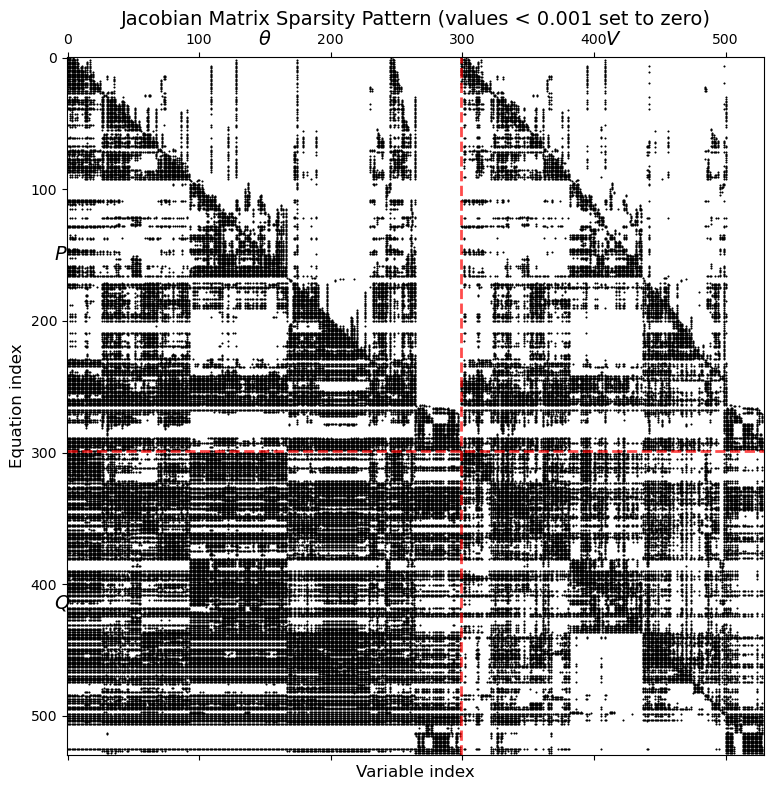
We can clearly see the four blocks separated by the red lines.
Visualizing System Voltages#
We use networkx
to visualize power network topology and power flow results for intuition.
This visualization approach:
Displays the network structure with buses (nodes) and transmission lines (edges)
Encodes bus voltage magnitudes through color mapping (red for low, green for high voltage)
Differentiates bus types using node sizes and colors (Slack, PV, PQ)
Shows power flow directions with arrows
Applies connected component sampling for large networks to maintain topological context
Selectively labels critical buses (generators and slack buses) to reduce visual clutter
The visualization provides intuition into:
System connectivity and topology
Voltage profile across the network
Generator locations and their impact on voltage support
Power flow patterns and potential congestion areas
Interactive Use
For interactive exploration in Jupyter notebooks, consider using tools like ipycytoscape
or networkx
with ipywidgets
to enable zooming, filtering, and displaying additional information on hover.
import networkx as nx
import matplotlib.pyplot as plt
import numpy as np
import time
def visualize_power_system(ss, ndata, max_buses=100, figsize=(12, 10),
sample_large_network=True, seed=42,
show_critical_labels=True, connected_sampling=True):
"""
Power system visualization with connected sampling and selective labeling.
Parameters:
-----------
ss : ANDES system object
ndata : NodalData object with voltage magnitudes and angles
max_buses : int, maximum number of buses to display (samples if larger)
figsize : tuple, figure size
sample_large_network : bool, whether to sample large networks
seed : int, random seed for reproducibility
show_critical_labels : bool, whether to show labels for important buses only
connected_sampling : bool, whether to use connected component sampling
"""
start_time = time.time()
print("Creating network visualization...")
# Create a new figure with axes
fig, ax = plt.subplots(figsize=figsize)
# First create a full graph to use for connected sampling
full_graph = nx.Graph()
# Add all buses as nodes
for i in range(ss.Bus.n):
if i in ss.Bus.idx2uid(ss.Slack.bus.v):
bus_type = 'Slack'
elif i in ss.Bus.idx2uid(ss.PV.bus.v):
bus_type = 'PV'
else:
bus_type = 'PQ'
full_graph.add_node(i, voltage=ndata.V[i], angle=ndata.theta[i], type=bus_type)
# Add all lines as edges
for idx, (from_bus, to_bus) in enumerate(zip(ss.Line.bus1.v, ss.Line.bus2.v)):
from_idx = ss.Bus.idx2uid(from_bus)
to_idx = ss.Bus.idx2uid(to_bus)
full_graph.add_edge(from_idx, to_idx)
# Determine if we need to sample the network
if ss.Bus.n > max_buses and sample_large_network:
print(f"Network too large ({ss.Bus.n} buses), sampling {max_buses} buses")
if connected_sampling:
# Start with slack buses and expand outward
slack_buses = list(ss.Bus.idx2uid(ss.Slack.bus.v))
if not slack_buses: # If no slack bus, start with any bus
slack_buses = [0]
# Get a connected component starting from a slack bus
try:
# Use BFS to get connected buses
bfs_edges = list(nx.bfs_edges(full_graph, source=slack_buses[0]))
sampled_buses = {slack_buses[0]}
# Add buses breadth-first until we have enough
for u, v in bfs_edges:
sampled_buses.add(v)
if len(sampled_buses) >= max_buses:
break
bus_indices = list(sampled_buses)
except nx.NetworkXError:
# Fallback to random sampling if BFS fails
np.random.seed(seed)
bus_indices = np.random.choice(np.arange(ss.Bus.n),
size=min(max_buses, ss.Bus.n),
replace=False)
else:
# Random sampling
np.random.seed(seed)
bus_indices = np.random.choice(np.arange(ss.Bus.n),
size=min(max_buses, ss.Bus.n),
replace=False)
else:
# Use all buses
bus_indices = np.arange(ss.Bus.n)
# Create display graph with only the sampled buses
G = nx.Graph()
# Add nodes (buses)
for i in bus_indices:
# Determine bus type
if i in ss.Bus.idx2uid(ss.Slack.bus.v):
bus_type = 'Slack'
elif i in ss.Bus.idx2uid(ss.PV.bus.v):
bus_type = 'PV'
else:
bus_type = 'PQ'
G.add_node(i, voltage=ndata.V[i], angle=ndata.theta[i], type=bus_type)
# Add edges (lines) - only those connected to sampled buses
for idx, (from_bus, to_bus) in enumerate(zip(ss.Line.bus1.v, ss.Line.bus2.v)):
from_idx = ss.Bus.idx2uid(from_bus)
to_idx = ss.Bus.idx2uid(to_bus)
# Only add edges where both buses are in our sampled set
if from_idx in bus_indices and to_idx in bus_indices:
# Use a simplified approach for power flow direction
angle_diff = ndata.theta[from_idx] - ndata.theta[to_idx]
flow_direction = 1 if angle_diff > 0 else -1
G.add_edge(from_idx, to_idx, direction=flow_direction)
print(f"Graph created with {G.number_of_nodes()} nodes and {G.number_of_edges()} edges")
# Set node positions using a faster layout algorithm for large graphs
if G.number_of_nodes() < 50:
pos = nx.spring_layout(G, seed=seed)
else:
pos = nx.kamada_kawai_layout(G)
print(f"Layout calculated in {time.time() - start_time:.2f} seconds")
# Define node sizes and colors based on type
node_sizes = []
node_colors = []
# Create labels dict - either critical buses only or all if small network
labels = {}
for n, data in G.nodes(data=True):
# Size and color based on type
if data['type'] == 'Slack':
node_sizes.append(150)
# Always label slack buses if showing critical labels
if show_critical_labels:
labels[n] = str(n)
elif data['type'] == 'PV':
node_sizes.append(100)
# Label generator buses if showing critical labels
if show_critical_labels:
labels[n] = str(n)
else: # PQ buses
node_sizes.append(50)
# Color based on voltage (using RdYlGn colormap)
v_normalized = (data['voltage'] - 0.9) / 0.2 # Range from 0.9 to 1.1
v_normalized = np.clip(v_normalized, 0, 1)
color = plt.cm.RdYlGn_r(v_normalized)
node_colors.append(color)
# If network is small and we're not doing critical-only labels, label all nodes
if G.number_of_nodes() < 50 and not show_critical_labels:
labels = {n: str(n) for n in G.nodes()}
# Compute layout
pos = nx.kamada_kawai_layout(G) # or kamada_kawai_layout(G)
# Separate nodes by type
slack_nodes = [n for n, d in G.nodes(data=True) if d['type'] == 'Slack']
pv_nodes = [n for n, d in G.nodes(data=True) if d['type'] == 'PV']
pq_nodes = [n for n, d in G.nodes(data=True) if d['type'] == 'PQ']
def get_node_colors(nodes):
return [plt.cm.RdYlGn_r(np.clip((G.nodes[n]['voltage'] - 0.9) / 0.2, 0, 1)) for n in nodes]
# Draw edges FIRST so nodes appear on top
nx.draw_networkx_edges(G, pos, ax=ax, edge_color='gray', arrows=False, width=1.0)
# Draw Slack (squares)
nx.draw_networkx_nodes(G, pos, nodelist=slack_nodes, node_color=get_node_colors(slack_nodes),
node_size=120, node_shape='s', ax=ax)
# Draw PV (triangles)
nx.draw_networkx_nodes(G, pos, nodelist=pv_nodes, node_color=get_node_colors(pv_nodes),
node_size=90, node_shape='^', ax=ax)
# Draw PQ (circles)
nx.draw_networkx_nodes(G, pos, nodelist=pq_nodes, node_color=get_node_colors(pq_nodes),
node_size=50, node_shape='o', ax=ax)
# Draw labels last
nx.draw_networkx_labels(G, pos, labels=labels, font_size=8, ax=ax)
# Add legend
slack_patch = plt.Line2D([0], [0], marker='s', color='w', markerfacecolor='gray',
markeredgecolor='black', markersize=10, linestyle='None', label='Slack Bus')
pv_patch = plt.Line2D([0], [0], marker='^', color='w', markerfacecolor='gray',
markeredgecolor='black', markersize=9, linestyle='None', label='PV Bus')
pq_patch = plt.Line2D([0], [0], marker='o', color='w', markerfacecolor='blue',
markersize=6, label='PQ Bus')
legend1 = ax.legend(handles=[slack_patch, pv_patch, pq_patch],
loc='upper right', title="Bus Types\n(marker shape)")
# Add title and turn off axis
ax.set_title(f"Power Network Visualization -- ({G.number_of_nodes()} buses)")
ax.axis('off')
# Create a separate colorbar for voltage levels
sm = plt.cm.ScalarMappable(cmap='RdYlGn_r', norm=plt.Normalize(vmin=0.9, vmax=1.1))
sm.set_array([])
cbar_ax = fig.add_axes([0.92, 0.1, 0.02, 0.3]) # [left, bottom, width, height]
cbar = fig.colorbar(sm, cax=cbar_ax)
cbar.set_label('Voltage (p.u.)')
print(f"Visualization completed in {time.time() - start_time:.2f} seconds")
return fig, ax
fig, ax = visualize_power_system(ss, ndata,
max_buses=999,
connected_sampling=True,
show_critical_labels=True)
plt.show()
Creating network visualization...
Graph created with 300 nodes and 409 edges
Layout calculated in 0.36 seconds
Visualization completed in 0.75 seconds
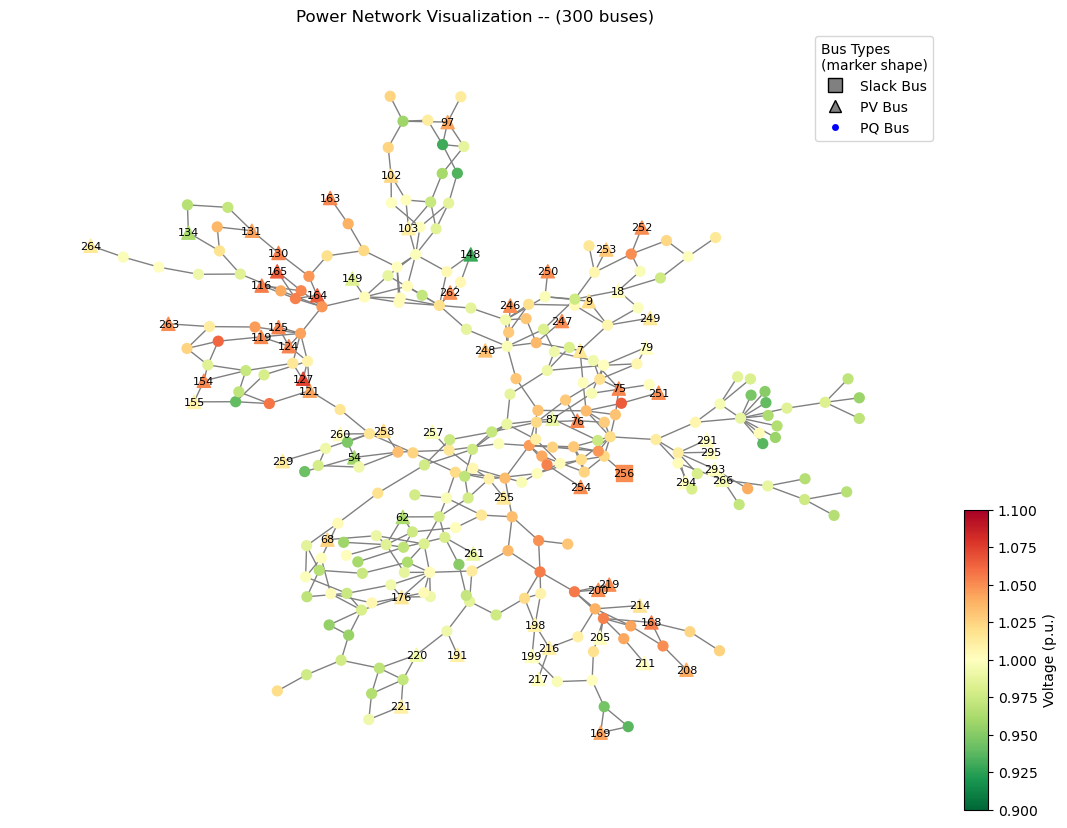